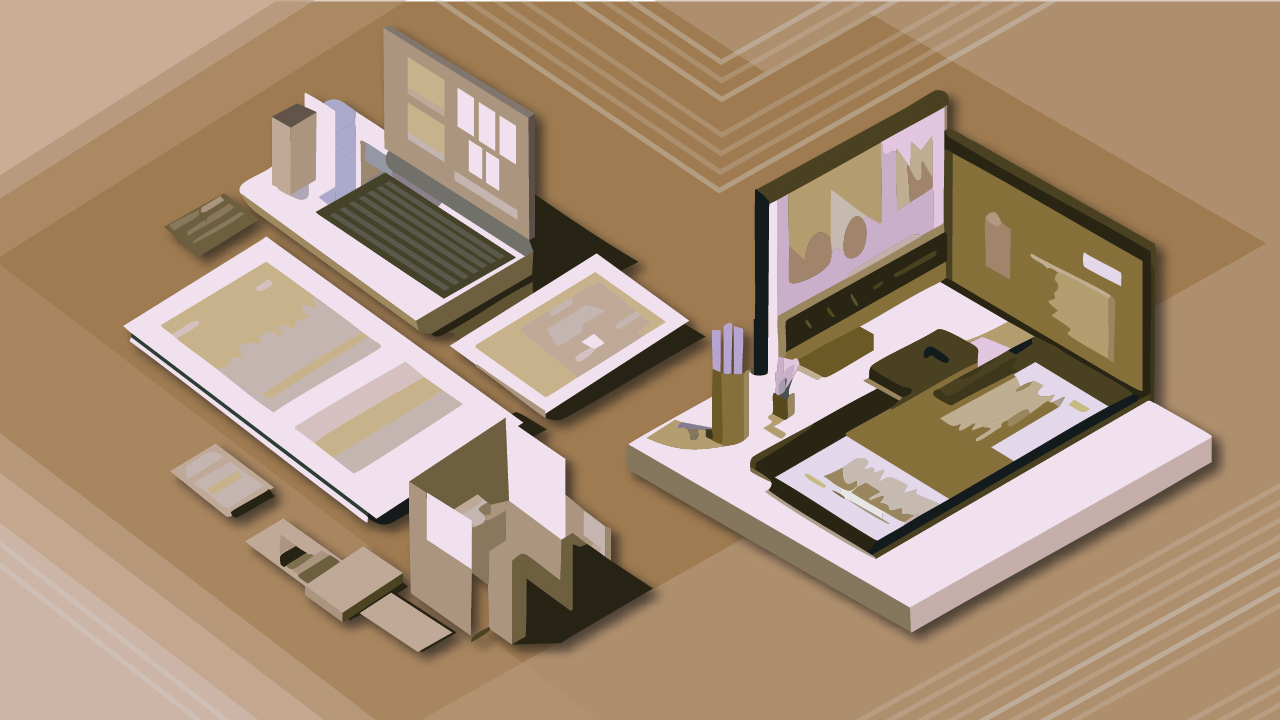
Let us understand in details, how this powerful combination of machine learning and data warehousing, enhances analytics and propels organizations into the realm of predictive intelligence.
1.Unified Data Ecosystem:Traditional data warehousing systems store and manage vast amounts of historical data. Machine learning models thrive on data, and the blend of these technologies creates a unified ecosystem where historical and real-time data seamlessly coexist. This unified data environment allows organizations to harness the power of both structured and unstructured data, enabling more comprehensive and accurate predictive modeling.
2.Predictive Analytics Unleashed:Machine learning algorithms are adept at identifying patterns and trends within large datasets. By integrating ML into data warehousing, organizations can move beyond retrospective analysis to predictive analytics. Predictive models can forecast future trends, customer behaviours, and potential business outcomes, providing a competitive edge by enabling proactive decision-making.
3.Enhanced Data Quality and Cleansing:Machine learning algorithms can contribute to data quality by identifying and correcting anomalies, outliers, and inconsistencies in the data stored within the data warehouse. By automating data cleansing processes, organizations can ensure that the insights derived from analytics are based on accurate and reliable data.
Practical Applications:1.Customer Segmentation and Personalization:
ML algorithms can analyze customer data within the data warehouse to identify distinct segments based on behaviour, preferences, and demographics. With this information, businesses can tailor their products, services, and marketing strategies to specific customer segments, leading to improved customer satisfaction and increased engagement.
2.Supply Chain Optimization:Predictive analytics powered by machine learning can optimize supply chain operations by forecasting demand, identifying potential disruptions, and streamlining inventory management. This proactive approach enhances efficiency, reduces costs, and minimizes the impact of unforeseen events on the supply chain.
3.Fraud Detection and Security:Machine learning models integrated into data warehousing systems can continuously analyze transactional data to identify patterns indicative of fraudulent activity. By detecting anomalies in real-time, organizations can enhance security measures and mitigate financial risks associated with fraudulent transactions.
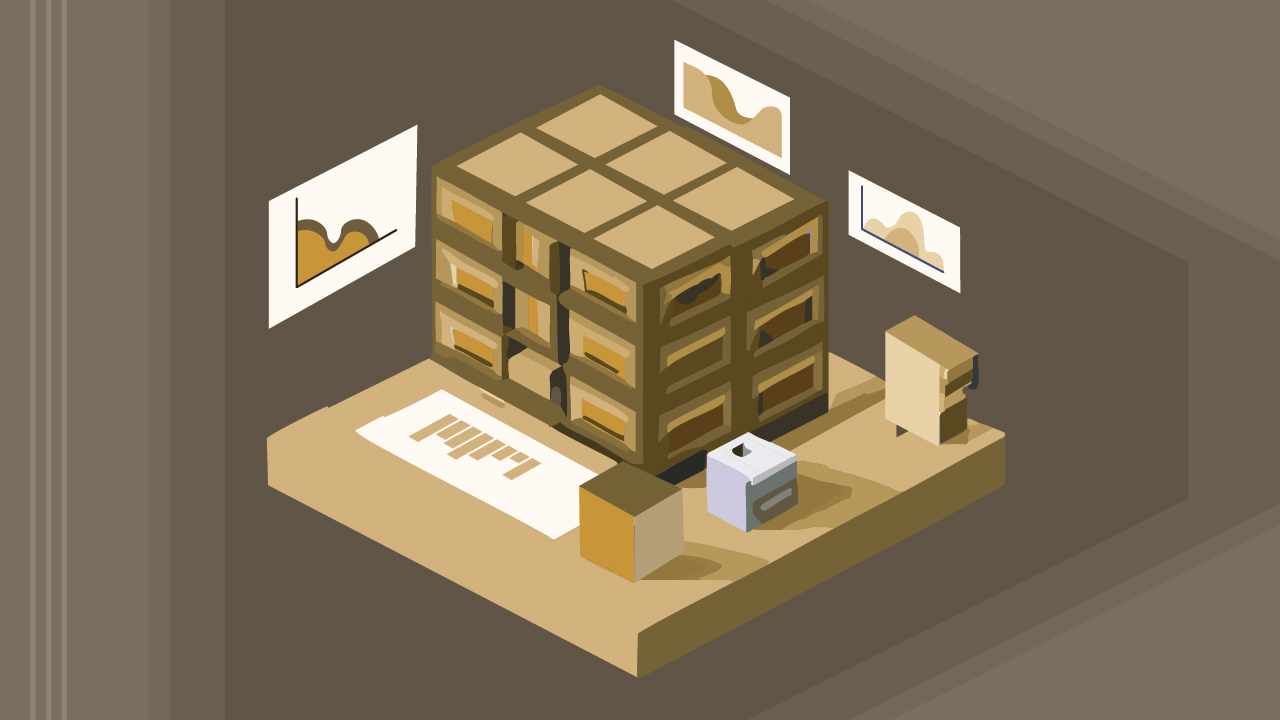
Challenges and Considerations:
While the integration of machine learning into data warehousing offers tremendous potential, it comes with its own set of challenges and considerations.
1.Data Governance and Privacy:Ensuring compliance with data governance policies and addressing privacy concerns become critical when dealing with sensitive information, especially as machine learning models may have access to a wide range of data.
2.Scalability and Performance:Scalability issues may arise as organizations deal with increasingly large datasets. Optimizing the performance of machine learning algorithms within the data warehouse is essential to maintain responsiveness.
3.Skillset and Talent:Developing and maintaining machine learning models requires a specialized skill set. Organizations need to invest in training or hiring data scientists and machine learning experts to maximize the benefits of this integration.
The convergence of data warehousing and machine learning marks a paradigm shift in the way organizations leverage their data for actionable insights. By embracing predictive analytics, businesses can not only understand past trends but also anticipate future developments. As the synergy between data warehousing and machine learning continues to evolve, organizations that harness the power of this integration will be better positioned to thrive in the data-driven era.
Author Name:-Antara Mukherjee
Author Email-Id:- antara.mukherjee@omfysgroup.com